As artificial intelligence (AI) adoption accelerates, business leaders face a tough decision: Do we want to scale our in-house unit or look into AI development outsourcing? Either approach comes with a unique set of operational challenges and cost drivers.
Key Factors That Impact In-House AI Development Costs
Payroll, research and development (R&D), project oversight, and ongoing maintenance will be key centers of in-house AI model development.
Talent Acquisition and Retention
An effective AI development team requires several core roles: data scientist(s), data engineer(s), and machine learning or AI engineers. Plus, you’ll need supporting staff like project managers to oversee execution and domain experts to contribute industry-specific knowledge for model training and validation. Yet, filling these positions is hard.
High demand and low supply have created competitive tension in the labor market. For almost 80% of tech leaders “insufficient skills and expertise” are a major bottleneck to rolling out AI. Over a quarter also say that AI skills shortages will become an even bigger issue next year.
Limited talent availability drives up the salary costs globally. In Q1 2024, median AI engineer salaries stood at:
- San Francisco Bay Area: $318,150
- Greater Seattle Area: $310,000
- Switzerland: $204,027
- United Kingdom: $148,700
- Israel: $148,275
- Canada: $115,349
- Australia: $113,064
- Singapore: $110,415
Median compensation for data science roles sits at $150K annually. Limited talent supply creates competition among employers. Tesla and OpenAI have been in a state of tug-of-war for AI engineers for several months, with each company raising base pay. Other businesses have also issued retention bonuses to prevent key staff from leaving.
Subsequently, it should come as no surprise that employee compensation accounted for 29% to 49% of the total cost of developing frontier AI models like GPT-3, OPT-175B, and Gemini Ultra 1.0, according to research from Epoch.ai.
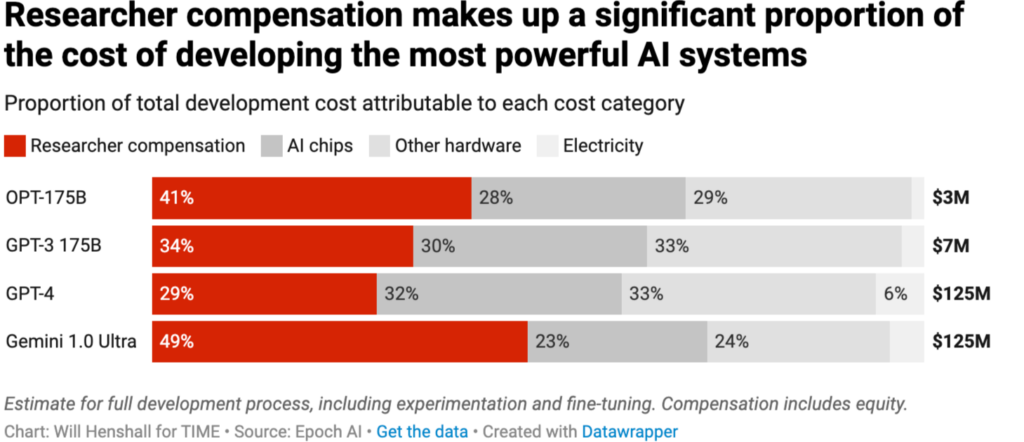
Research & Development Costs
AI model development is tedious, data-heavy, and error-prone. Most data science teams end up shelving over 80% of models before deployment. The most common reasons for abandoning candidate models include subpar model performance on production data, technical implementation issues, and integration challenges with legacy architectures.
For inexperienced project managers, such issues are often hard to foresee (and budget for accordingly), leading to higher overall execution costs. Even when models do get released to production, that’s only the start of the journey. Teams may encounter additional challenges (and costs) related to supporting cloud infrastructure management and optimization, namely around scalability, security, monitoring, and maintenance.
Productized AI and ML solutions are prone to drift – a progressive decay in performance that happens when either the input data or the task the model was trained to do changes over time. If left undetected, model drift can cause significant financial impacts. Zillow lost over $500 million to an error in its proprietary property valuation algorithm that used 30-day-old data for real-time decisions.
Overall, 93% of ML engineers encounter various issues with their production models daily/weekly, which further increases the cost of AI model development.
Observability and Maintenance
Ongoing AI model monitoring is crucial to avoid costly mishaps and ensure its topmost security. You’ll need to set up an observability platform to track key model performance metrics. Model errors, biases, and unexpected outputs can create major real-world risks. EdTech company iTutor Group had to settle a lawsuit after its AI-powered recruiting software unfairly rejected certain groups of job applicants.
With emerging AI regulations, the focus on model explainability and transparency will grow stronger. Hence, it’s important to invest in the right technology for monitoring productized AI and ML models and implement extra process controls to minimize AI risks and promote continuous model improvement.
Finally, you should also factor in the total infrastructure costs, which cover your cloud bill and APIs and/or licenses for deployed models. Beyond computing resources, most AI and ML models also require load balancing, networking services, telemetry platforms, continuous integration tools, and container services. When left unchecked, these costs can spiral out of control. For a gaming startup, maintaining a Gen AI model for its gameplay costs about $200,000 a month. High infrastructure bills can make it hard to demonstrate the long-term ROI of AI.
Project Overheads
To arrive at a fair number, you also need to factor in miscellaneous project overheads that include the involvement of supporting roles like:
- Project and/or product managers who oversee the model roadmap, development process, resource allocation, and timelines. Their salaries are a significant overhead cost.
- Administrative staff like HR professionals, accounting, and other professionals who support AI development teams.
Likewise, there are also other operational expenses related to personal hardware, software tools and licenses, office space, and any other perks your in-house teams have.
Key Factors That Influence Outsourced AI Development Costs
The cost of AI development outsourcing will be mostly driven by the vendor’s service model, project scope, and your ability to ensure effective collaboration.
Vendor Service Model
There’s no shortage of companies offering machine learning and artificial intelligence outsourcing services. You should conduct baseline due diligence, covering vendor’s reputation, technical capabilities, team competence, security practices, and overall software development practices. Pay special attention to the proposed AI/ML services models as these will impact the project costs most.
AI and machine learning service providers typically offer one of the following service models:
- Consulting services: Strategic advisory on AI implementation, which may include maturity assessments, risk maps, technology road mapping, and business-level guidance on how to integrate AI into your operations best. However, consulting services don’t always assume hands-on help with model development.
- Managed services: Support for conceptualizing, implementing, and managing AI models, along with solution maintenance. It’s a good service model for long-term collaboration, allowing you to hand off all AI-related processes to an expert external team.
- Development centers offer a more flexible service delivery model, where services are pre-agreed and costs are based on the scope of work (SoW). This model also allows for dynamically adjusting the team’s involvement, making it ideal for smaller, short-term projects.
- Team extension. Gain the missing AI, ML, and data engineering skill sets for your team to accelerate the model development processes.
Compared to in-house development, businesses can gain substantial savings on talent acquisition and payroll costs with AI outsourcing. However, the benefits span well beyond "cost savings" since you also gain access to new capabilities and stronger expertise in successfully deploying AI and ML models. Additionally, you gain extra operating agility. Many service models allow you to dynamically adjust the scope and scale the teams to respond faster to new market opportunities.
Project Scope and Complexity
Project specifications will largely dictate the exact costs. When evaluating the costs associated with outsourcing AI projects, you should factor it:
- Model complexity. Simple binary classification models will require less resources and expertise to develop than, for instance, training an AI agent with reinforcement learning or developing a custom large language model (LLM).
- Model type. Fully custom AI systems – built specifically for your business using a combination of open-source and proprietary technologies – generally cost more to develop but less to maintain in the long run. Pre-built AI as a service (AIaaS) requires less initial configuration but can accumulate a higher TCO over time. Some options may also have limited customization capabilities or scalability potential.
- Infrastructure configuration. This includes spending on compute, storage, networking, logging, and monitoring software on your end, plus the time the vendor spends building and maintaining it. Integration and deployment challenges due to inefficiencies in existing systems can increase the latter.
- Data requirements. The quantity and quality of data needed for your use case can significantly impact costs. Often, a smaller model (with fewer parameters) trained on high-quality data can be more efficient than a larger one trained on limited data. You may also need to change the current data management infrastructure to accommodate the model requirements (e.g., create real-time data pipelines to feed data to the new AI model).
Generally, most AI development projects are interactive in nature. It makes sense to start with a proof of concept (PoC) deployment to test the initial hypothesis, validate the selected model training approaches, and troubleshoot possible issues. Then develop a product roadmap, specifying additional features and product scaling pathways to accommodate more users. This way, you can reduce sinking costs into unproven ideas while also keeping a healthier cash flow.
Lastly, when it comes to Gen AI model development, fine-tuning existing models (e.g., GPT, LLaMA, DALL-E, etc) is the optimal approach to maximizing the benefits without ballooning the costs. Gen AI model fine-tuning assumes re-training an existing model to perform a specific task. For example, generate answers based on a provided financial dataset. For example, fine-tuning a GPT model using Azure OpenAI can cost from $34 to $68 per compute hour depending on the model used. Afterwards, the cost to run a model will be between $1.70 to $3 per hour.
Communication and Collaboration
The final important cost driver in AI outsourcing is your ability to build an effective collaboration process with an external team. According to Rand Research, the top root cause of AI project failures is industry stakeholders often misunderstand — or miscommunicate — what problem needs to be solved with AI.
This leads to incomplete project specifications, subsequent delays, and costly reworks. Likewise, many companies emphasize the technology itself (i.e., implementing AI) rather than focusing on an actual problem the technology can solve. Some also don’t account for existing AI limitations, failing to assess the feasibility of set requirements.
A great AI development partner would highlight the gaps in your strategy and suggest mitigation strategies or alternative use cases. However, your company should be open to listening and acting upon such feedback. This requires a high degree of trust and mutual accountability, which takes time to cultivate. Being transparent about your expectations, receptive to feedback, and available for joint strategic sessions with your team reduces the odds of negative outcomes like scope creep, project delays, and increasing costs.
Artificial Intelligence Outsourcing or In-House Development: Which Option is Better?
AI implementation is an inherently complex, requiring cross-functional capabilities and, often, broader changes to the operating model. Building an in-house unit gives you the advantage of cultivating proprietary know-how. However, this approach also requires more investments in bringing and nurturing your talents.
Outsourcing data and AI projects to an external technology partner reduces the costs associated with AI talent acquisition, retention, and management. An experienced partner also brings their ‘baggage’ of knowledge from past projects — recommended frameworks, toolsets, and accelerators for effectively solving various AI model development lifecycle issues.
Subsequently, you can increase the time-to-market for AI solutions to improve your operating efficiencies and profitability. Svita Systems has helped healthcare companies deploy an AI-powered medical coding solution for anesthesia procedures, which improved the overall efficiency of the healthcare revenue cycle. A global hotel aggregator platform, in turn, launched AI-powered demand forecasting capabilities and a new product recommendation engine for its customers with the help of our engineering teams, which brought substantial revenue growth.
If you’re looking for a great balance of expertise, agility, and cost-efficiency, Svital Systems AI and machine learning development services may be the optimal choice for your business.