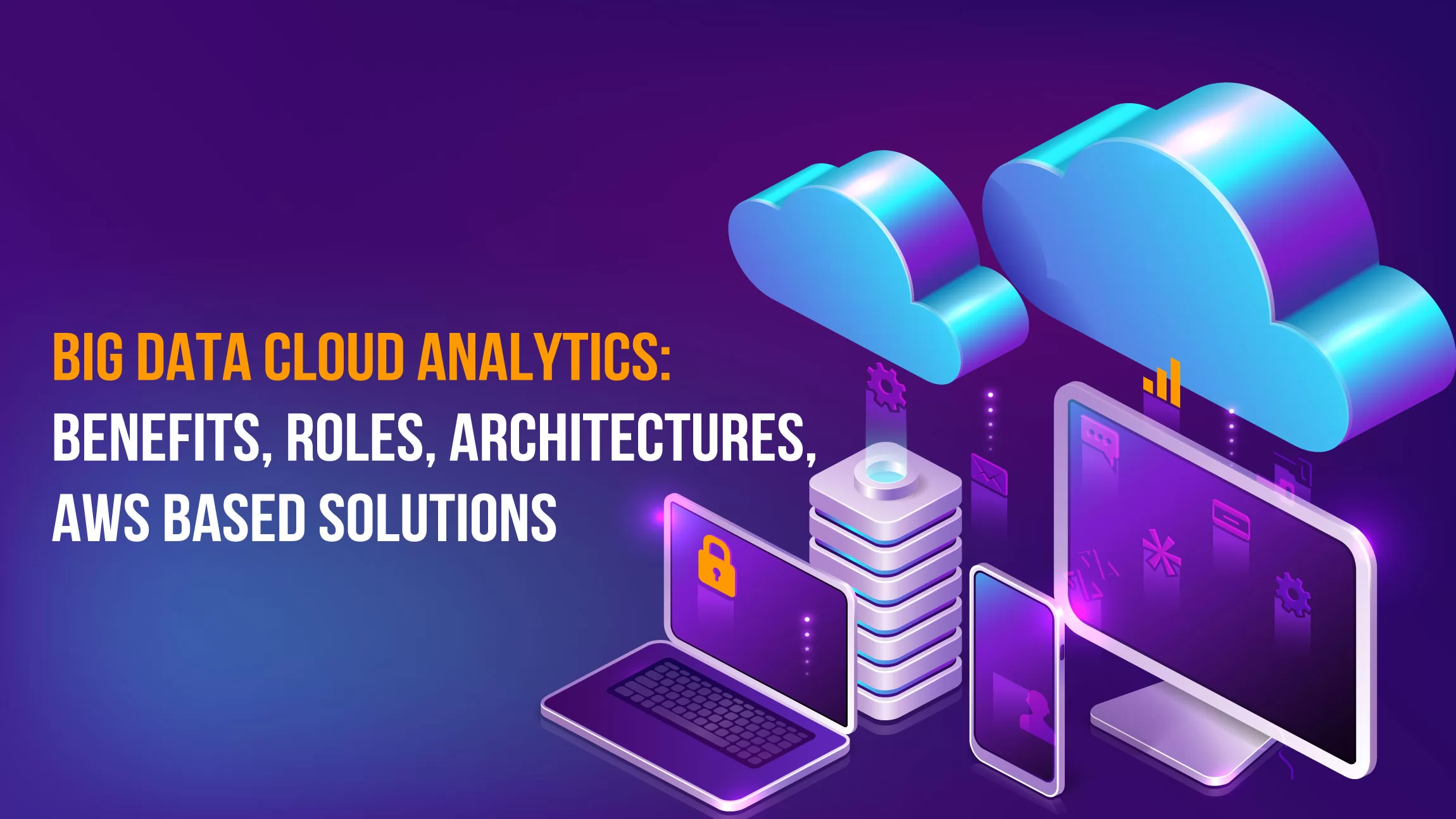
Big Data Cloud Analytics: Benefits, Roles, Architectures, AWS based solutions
For years, organizations have leveraged the power of data analytics to power decision-making tasks that help maximize profits. In a perfect world, data analytics helps minimize or completely eliminate a lot of the guesswork involved in trying to figure out what consumers want or what their major pain points are. In the real, albeit not as perfect world, data analytics is pretty good at enabling the systematic tracking of data patterns to build intelligent and fact-based insights that help strategize for the future, the right business approach to use, and the tasks that need to be carried out to reduce uncertainty.
Data analytics is also a great ally to detect what might attract prospective customers by recognizing patterns and establishing a course of action.
As the world continues to evolve, especially with the COVID-19 pandemic still raging on, analytics is a safe spot that gives organizations a competitive edge in identifying changing business conditions to adapt accordingly and take prompt action.
Now, let’s add cloud computing into the mix. Cloud computing helps organizations be more effective, agile, and responsive when it comes to executing business processes. By combining both data analytics and cloud computing, organizations can unlock the power to store, analyze, and process big data to meet modern business demands.
Within this context, it’s inevitable to talk about Amazon Web Services (AWS). AWS is the most comprehensive suite of analytics services that accommodate data analytics tasks including data storage, data movement, data lakes, big data analytics, streaming analytics, machine learning, log analytics, and everything in between.
But before we get too excited with AWS as it truly is the one-stop shop for all things related to cloud analytics, let’s talk about the business benefits of cloud analytics and the roles needed.
Spoiler alert, you will find lots of AWS tidbits throughout the piece.
What are Big Data Cloud Analytics Platforms and what are the business benefits of using one?
Before we dive into the Big Data Cloud Analytics platforms available, let’s first shed some clarity on what cloud analytics is. If you take into account that cloud computing is a collection of software and hardware elements that can be accessed remotely via web browsers, cloud analytics is the practice of applying analytics principles to the information that moves through cloud computing elements on cloud drives. Now, that explanation may ring a bell because it’s kind of similar to the one of Data Analytics, and you wouldn't be wrong. But there are key distinctions between the two that make them strikingly different.
For starters, cloud analytics solutions revolve around the centralization of resources as a service, all delivered from a data center. Resources include servers, networks, bandwidth, storage, operating system, software, and more. In turn, data analytics is the practice of analytical modeling or data preparation for the adequate processing of data for quantitative analysis. Data analytics comes in handy when trying to extract insights from information to drive improvements, understand trends, and boost performance.
As stated earlier, nearly every organization is moving to the cloud, which is why cloud analytics tools have taken on a higher meaning. In fact, key industries such as medicine, automotive, gaming, and more, are leveraging cloud analytics services as their de facto framework to yield actionable insights.
For example, in gaming, analytics is key to track trends, diagnose problems, and improve game design. With a deeper perspective into what works and what doesn’t work in a game, game developers and designers can quickly make changes to drive user retention and improve user experience. Another example of cloud analytics in the gaming industry is the use of predictive analytics which help forecast or anticipate player actions to give a competitive advantage. By ingesting historical and current data, predictive analytics can help influence purchase decisions, optimize lifetime value, and prevent churn.
There are clear benefits of cloud analytics, but there are also quite a few big data analytics challenges as well, which will continue to exist as big data cloud analytics is a massive field that continues to grow and evolve. For instance, some of the key benefits cloud analytics include:
- Reduction in operational costs. Let's face it, housing your own on-premises system involves a hefty investment that includes IT headcount, development, hardware, servers, and more. With cloud analytics, you lower the costs by eliminating the need for those elements.
- Scalability. A word that gets thrown around a lot, but that’s particularly true in cloud analytics. Cloud-based frameworks provide on-demand capabilities that are flexible and virtually limitless. Available resources are easy to scale up or down, without having to pay upfront or provide maintenance to them.
- Agile. Significantly faster data processing that enables organizations to be nimble and responsive to changing business demands.
- Greater sharing and collaboration capabilities. The cloud was designed to be the ultimate sharing space, where everyone can access the same information, files, data sets, and so on, and share with a few simple clicks.
- Remove silos. Thanks to a unified and centralized system, the cloud makes it easier to collaborate between different teams, which directly creates better communication streams where teams have access to more insights across departments.
- Better security. With no local hard drives to steal, the cloud is inherently more secure than any other means of data storage. With cloud analytics, data is regularly backed up to servers in different locations and sensitive data is not shared with unsafe hardware elements like flash drives. Data is typically password-protected where only folks who need to view the data are allowed to, and there are audit logs to track what, when, and what users did with what they accessed.
And because not everything is rainbows and sunshine, here are some of the most prominent big data analytics challenges:
- There’s still a shockingly high number of people who don’t trust the cloud. Some organizations fear putting all their data in the “hands” of someone else, and controversies around the transfer of personal data don't help the case as there have been major data breaches in the headlines that put people at great unease. For example, financial institutions are especially wary of keeping sensitive information in the cloud.
- Complexity. Because the dispersion area of sources is greater thanks to the many different data formats and owners, cloud migration processes can become a complete headache. For example, you can have Excel data, SQL databases, PDFs, JPEGs, PNGs, and more, making your data inconsistent, ambiguous, and messy to work with. To remove some of the complexity in cloud analytics, it’s important to clean, process, and integrate data prior to any analytics task being performed.
- Too big. Data volumes can easily surpass the biggest number we can think of. Cloud-based analytics solutions should be optimized to deal with these massive amounts of data, such as data warehouses or data lakes.
How to use big data analytics for business in your company
As businesses continue to move entire workloads and legacy data to data warehouses or analytical databases to the cloud, cloud computing grows more critical. If your organization is already leveraging cloud analytics or it’s about to embark on this exciting journey, here are some of the best tools based on the department they focus on that you can use to get the most out of your analytics efforts.
- Sales Analytics. Cloud analytics tools that focus on sales are typically designed to help you manage customers and prospects, in a unified view where you can also assess sales in all the geographies your business operates, as well as monitor how the sales team is doing in terms of closed and ongoing deals. By taking a hard look at these numbers, you can get clarity on important trends, what can be improved, and gaps in the sales funnel, to name a few.
- Financial Analytics. Beyond traditional financial methods, financial analytics tools help businesses extract valuable insights about revenue and expense patterns so you plan better budgets, allocate resources more strategically, and protect the overall financial wellbeing of your organization.
- Website analytics. Looking to analyze your website traffic? Then website analytics is the solution. Tools that focus on website analytics help you make sense of the conversion rate of your website, traffic, bounce rates, and more, so you can assertively adjust the user experience in the spirit of increasing profitability and revenue.
- Social media analytics. With social media analytics tools, you can collect and interpret social media engagements and interactions. With deeper examination of social media activity, you can quantify how to best distribute and invest your social media budget and resources, or create the right campaign for a specific audience.
Of course, there are more categories of tools you can use for cloud analytics, and even within the ones we just describe, you can find sub-categories of specialized software that, in a nutshell, help you make sense of data so you can make smart decisions, be it through simple or more advanced analytics technologies, such as AI and machine learning.
Another thing to keep in mind is that not every organization is at the level where they can have in-house resources to maintain analytics systems. Luckily, there are services such as Amazon Web Services (AWS) that provide affordable solutions to meet those demands without breaking the bank, which we’ll explore in the next section.
What talents does a business need to benefit from data analytics?
But before we go into that, we’d like to first focus on what it takes to build the right teams for cloud analytics consulting or services, whether they’re in-house or outsourced. Here’s a breakdown of the roles needed:
- Cloud Analytics Manager. Kind of obvious, right? A cloud analytics manager is in the midst of it all, in charge of contributing and delivering strategy and vision for analytics, building the roadmap that looks after the budget and resource planning, as well as measuring performance.
- Chief Data Officer. This impressive role has the responsibility of executing the entire data analytics strategy, all with the goals of boosting quality, reliability, and accessibility. They also go by Chief Data and Analytics Officer, Chief Analytics Officer, and Chief Digital Information Officer.
- Data Architect. Also known as the Information Architect, this role is responsible for making information available and sharing it as necessary to make better, smarter business decisions. This role understands the impact of different analytics scenarios at an architectural level.
- Analysts. Within the spectrum of the role, analysts work with different use cases and scenarios, which in turn determines the skills and responsibilities needed for each. For example, some analysts require a statistical background while others may need more business intelligence background.
- Project managers. In charge of the end-to-end lifecycle of a project, these managerial roles are in charge of the successful implementation of all tasks within an analytics project.
- Data Engineer. This type of engineer focuses on making data understandable to all. They build, manage, and streamline data funnels for data use cases, including data cleaning, pre-processing, processing, and interpretation.
- Data Scientist. Once data is ready to be analyzed, data scientists use different modeling techniques to discover insights via algorithms, statistics, and visualization approaches.
- AI/Machine Learning Developers. The experts of intelligent technologies, these specialists make sure to embed applications with the right mix of algorithms, optimization, natural language processing, image recognition, and more, to detect patterns, enrich results, and more.
On-Demand Big Data Analytics on AWS
Big data analytics on cloud is critical as we continue to move to a more digital, interconnected environment, where the amount of data surpasses anything a human could possibly digest.
As stated earlier, these massive amounts of data can translate into meaningful insights if exploited correctly. Take business profiling, for example. With enough data about what products consumers are loving, how they’re using them, which pain points are they trying to solve with that product, how relevant they really are for their day-to-day life, and more, companies can easily glean information about which elements of their consumer strategy to focus on, create highly targeted audiences, or even generate new features that add value to a product in the eyes of the consumer.
To get to that granular level of insights, you must keep in mind that cloud analytics is not a senseless exercise to process huge amounts of data. No, it’s also important to discern which data points to use as extraction areas, where to collect the most valuable data, how to store it in the most affordable way possible, and then and only then, how to best analyze it to really make a difference and gain a profound competitive advantage.
Thanks to the robust and varied services that AWS offers, you can easily build and scale big data applications. Something that wasn’t entirely achievable a few years ago but that is now at your fingertips with enough training and domain of specific tools.
Whether you’re building an app that needs batch processing or real-time streaming, AWS is your ally. Next, we’ll describe some of the most prominent AWS analytics tools you can use for your app projects.
Amazon Athena
Interactive and serverless in nature, Amazon Athena is a data analysis tool that processes complex queries. This query service is a top choice to analyze data paired with Amazon S3 (which we’ll focus on next) via standard SQL. Thanks to its serverless approach, Athena removes infrastructure out of the equation so it becomes easier for users to manage and to only pay for the queries they are actively running.
Not to be confused with a database, Athena lets you point data to Amazon S3 and determine the schema needed so you can begin querying all you want using SQL. Launched in 2016, Amazon Athena complemented the already growing list of cloud analytics services that AWS offered. In a matter of a few clicks, Amazon Athena users are well under way to leverage data stored in Amazon S3, run queries, and get results in seconds.
Another great aspect of Amazon Athena is that it scales automatically as it executes complex queries on large data sets. To this day, many still compare Amazon Athena with Microsoft’s SQL Server, but there are clear differences that set them apart. For example, Amazon Athena works best for data manipulation language (DML) queries operations on the database, while SQL Server is used for DML, as well as transaction control queries, data definition language, and more.
Amazon Athena was the answer to the market’s need for analytics tools for unstructured, semi-structured, and structured data stored in Amazon S3.
Amazon S3
As of the third quarter of 2021, the most popular vendor in cloud infrastructure services is no other than AWS, which holds a 32% share of the entire market closely followed by rivals Microsoft and Google.
One of the flagship offerings of AWS is Amazon S3, which stands for Simple Storage Service (S3, get it?) Back in 2006, AWS launched Amazon S3 on Pi Day (March 14, 2006, if you were wondering), making it the first AWS service available to the general public.
Amazon S3 is used to store and retrieve data at any time, any place, in any given amount, giving users the ability to tap into a highly scalable, quick, reliable, and budget friendly data storage service like no other. Amazon S3, as it continues to evolve, now includes easy management features to help users organize data for websites, manage costs, reduce latency, data backups, mobile applications, data restoration, and a multitude of other applications.
Before Amazon S3 came into the picture, it was incredibly challenging to find, store, and manage data, even though the volumes were a lot smaller when compared to what we’re currently dealing with. There’s a lot of storage involved in hosting high-traffic websites, running multiple applications at once, backing up files, and more, so it was critical to come up with a solution that served as an organization’s repository at an affordable price.
Thanks to the Amazon S3 object storage service, consumers can store and recover data with ease, accessing a highly scalable service that is designed to provide 99.999999999% durability and 99.99% object availability.
Regardless of your company size or industry, you can store and protect your data for any use case, including cloud-native applications, data lakes, and more. Amazon S3 offers different storage classes that meet unique business needs. For example, you can use the S3 standard class to store critical data for frequent access and save infrequently-used data in the S3 Standard-IA class.
Amazon EMR
Amazon EMR, also known as Amazon Elastic MapReduce, is a managed cluster solution aimed at streamlining the way big data frameworks are run, including the likes of Apache Hadoop, Apache Storm, Apache Hive, Presto, and Apache Spark. Amazon EMR runs large-scale distributed data processing jobs, machine learning applications, and interactive SQL queries leveraging open-source analytics.
For the most part, Amazon EMR is targeted at performing big data analytics, building scalable data pipelines, processing real-time data streams, accelerating data science and machine learning adoption, and moving large data sets in and out of other AWS databases and data stores including Amazon S3 and Amazon DynamoDB.
Just like Amazon S3 is the most popular storage infrastructure for a data lake, Amazon EMR is the de facto solution to run computing jobs while simultaneously storing data clusters in Amazon S3. Run as many clusters as you need, switch them off, or automatically resize them to scale up or down as needed.
The best use cases of Amazon EMR include the deployment of distributed data processing frameworks and decoupling compute and storage services for better resource utilization. Keep in mind that EMR is priced per second and only for the cluster resources you use.
Amazon Redshift
Amazon Redshift is a highly scalable, fully managed data warehouse offering from AWS. Amazon Redshift uses SQL to analyze structured and semi-structured data across operational databases, data warehouses, and data lakes thanks to AWS-designed hardware and machine learning there to optimize costs, performance, and scalability.
Amazon Redshift consumers rely on the service offering as a means to analyze gazillions of data and run complex analytical queries, making it by far, the most widely adopted data warehouse out there. In just a matter of seconds, users can run and scale analytics on all of their data, effectively managing their data warehouse infrastructure beyond what any other supplier offers.
The exabyte-scale data warehouse solution has analytics at the core of its design and is based on PostgreSQL8, and it shows as it has a column-oriented database aimed at connecting SQL clients and business intelligence tools.
Amazon Redshift data warehouses contain a collection of nodes that are assembled in what’s called a cluster. Each cluster runs its own Redshift processing and it includes at least one database. One of the biggest differentiators of Amazon Redshift is its speed, as it delivers query speeds on large data sets at an incredibly fast pace, which is virtually impossible to attain by means of a traditional data warehousing solution.
Amazon Kinesis
Amazon Kinesis is a best-in-class AWS service offering designed for data streaming analytics pipelines that make it easy to capture, process, and store video streams for analytics and machine learning.
A kinesis data stream is what’s known as a set of shards where each shard contains a sequence of data records. Those data records have a sequence number assigned by Kinesis Data Streams. Solely focused on performing analytics tasks on streaming data, AWS Kinesis is designed for quick Extract, Transform, Load (ETL) to swiftly capture and process streaming data, and then glean insights in real-time, including machine learning query application.
Amazon Kinesis lays the foundation to build and run apps in the cloud using Apache Flink as it’s designed to run in common cluster configurations, scale easily, and run at in-memory speed.
As a tool, Amazon Kinesis works for companies of all sizes that need help managing and integrating data across multiple platforms. For example, Netflix uses Kinesis to process terabytes of lgo data on a daily basis.
Amazon DynamoDB
Fast, flexible, NoSQL database. Those are the most frequently attached words when talking about Amazon DynamoDB.
This AWS service offers single-digit millisecond performance at any scale, giving apps a consistent performance with virtually unlimited throughput and storage, as well as automatic multi-region replication.
Amazon DynamoDB is a fully-managed, serverless, key-value NoSQL database that runs high-performance apps at all scales with built-in security, ongoing backups, in-memory caching, data export tools, and more. It’s typically used to develop software applications from scratch, create media metadata stores, deliver seamless retail experiences, and scale gaming platforms, to name a few.
Amazon DynamoDB offers important advantages over its competitors, the NoSQL database management systems like Apache Cassandra and MongoDB. From a more streamlined setup process, strengthened AWS security, and lower costs, Amazon DynamoDB integrates seamlessly with other AWS services which is particularly beneficial for organizations that are already leveraging AWS.
Amazon Elasticsearch
Amazon Elasticsearch is an industry leader in managed services aimed at deploying, operating, and scaling Elasticsearch in the AWS Cloud. Elasticsearch is an open-source search and analytics engine that helps organizations perform real-time app monitoring, click stream analytics, and log analytics, to name a few.
Amazon Elasticsearch packs all the resources needed for a cluster and it automatically detects and replaces any nodes that are not performing correctly, reducing the overhead that comes with self-managed infrastructure and Elasticsearch software.
By using Amazon Elasticsearch, consumers can directly access the Elasticsearch open-source API so they can work seamlessly with existing code and aps that are already leveraging Elasticsearch environments. The service offering also comes with built-in support for Kibana for easy visualization and data analytics.
Now, AWS introduced the successor of the Amazon Elasticsearch service and it’s called Amazon OpenSearch Service where users can search, visualize, and analyze petabytes of unstructured data.
Amazon OpenSearch is open-source and offers distributed search and analytics derived from Elasticsearch, supporting 19 versions of it, as well as visualization capabilities powered by OpenSearch dashboards and Kibana.
Namely, Amazon OpenSearch is used to monitor and debut apps and infrastructures, manage security and event information, and achieve a seamless, personalized search experience.
Amazon Lambda
Serverless compute service that lets users run code without controlling or provisioning servers. Amazon Lambda leverages highly-available, elastic infrastructures to run code, achieving compute resource administration tasks, including automatic scaling, capacity provisioning, server maintenance, and more.
As a serverless model, Lambda runs functions on a need-to basis, and you only pay for the compute time you use, which makes a great business case to reduce costs as you won’t be charged for code when it’s not run.
Amazon Lambda is event-driven, letting you run code for any type of app or backend service without the need for a server. Typical use cases of Amazon Lambda include processing data at scale, running interactive web and mobile backends, scaling APIs, enabling powerful machine learning insights, and creating event-driven applications.
As a whole, AWS provides one of the broadest (if not the most) sets of managed services for cloud analytics and data lakes, along with the biggest community of partners to help build applications from scratch. AWS competencies span data analytics, NoSQL/NewSQL, data integration and preparation, business intelligence, data visualization, data governance, and data security.
With AWS taking a bigger chunk of the cake when compared against its competitors, we continue to see a rise in the number of AWS-based roles in the IT industry. Now, specialized engineering roles are required to master at least AWS service offerings, with roles such as AWS Cloud Engineer, AWS Developer, AWS Solutions Architect, AWS DevOps Engineer, and AWS DevSecOps.
Conclusion
We said it before and we’ll say it again, everyone needs to be on the cloud or have a cloud strategy in the next decade or so, aided by the fact that the cloud is irrevocably becoming the place to be when looking for the most secure, reliable, and most importantly, affordable solution for data analytics in cloud computing. We envision a future where most organizations will save and process all of their data in the cloud, so everyone can access it from anywhere in the world, at any time.
When it comes to processing and analyzing large datasets, the need for impressive compute capacity is imperative. In this context, big data analytics is best suited for subscription-based models like the pay-as-you-go cloud computing model that AWS is so well-known for, where applications can easily scale up or down, on demand, without breaking the bank. The AWS model recognizes that business requirements change from one moment to the next, putting consumers in the driver’s seat so they can easily resize their environment without having to strategize for hardware or pouring more budget into acquiring enough capacity.
To meet the modern business demands of a cloud-driven environment, you should partner with coud-driven providers who are more than well acquainted with the latest developments and technologies surrounding cloud computing and cloud analytics. At Svitla Systems, we have perfected our cloud analytics craft for years and can safely attest to the expertise and value that we deliver to our clients. If you’re looking for the right cloud analytics partners in integration, data analysis, and consulting services, then please reach out to us so we can help you pick apart, advise, and provide the best assistance to your cloud analytics efforts.
Let's discuss your project
We look forward to learning more and consulting you about your product idea or helping you find the right solution for an existing project.
Your message is received. Svitla's sales manager of your region will contact you to discuss how we could be helpful.