2017 is just starting, and according to multiple sources, “big data” hype will be one of those trends that will be driving technology development. In this article, we are going to discuss what is important when working with big data and why having just data and tools is not enough.
Big data is usually identified by following criteria also known as 3V:
- Volume. Or quantity of stored data. The more data we have, the more potential insight we can generate from it. There is no predefined limit for minimal records volume to be considered big data, but usually it is millions or even billions of samples.
- Variety. Big data does not come in the same format. Generally speaking, it is just any information in any format that may help us to get insight in some area. It may be images or log files text data.
- Velocity. Or speed at which new data is generated. This is critical because our system must have enough storage and processing power to continue generating valuable insights while the amounts of data grow.
The key factor that identifies big data is that it uses big amounts of data to reveal hidden relations and dependencies between variables that are not connected by any laws and are coming from different sources. This relations and dependencies can reveal insights, which may help businesses to operate in a much more efficient way.
From an architecture perspective, a big data system contains the following components:
- Data lake. Or a place where all data is coming from, various sources stored in original format, such as files.
- Big data processing. Or technics and frameworks that extract meaningful facts from Data lake. This can be done only by a human being, as somebody needs to identify what particular facts you are looking for.
- Visualization and business intelligence. This system is using data from the results of the big data processing to make decisions.
So as we see from the items listed above, big data are just numbers and values, and without proper technics, it is just a “digital graveyard.” The need for storage grows dramatically, because more and more companies start to collect more “digital footprints” of their users, hoping that someday they will reveal hidden gems, insights that will improve their business. Collecting data without processing makes no sense that is why technics for data processing are important.
Unfortunately, machines cannot understand what insights you are looking for, that is why a new profession entitled a “data scientist” was created. Data scientists are like hunters, who initially define what insights they are looking for and use data processing technics and tools to distill a quantitative result from big data into something (be it words, pictures, charts, etc.) that everyone can understand immediately.
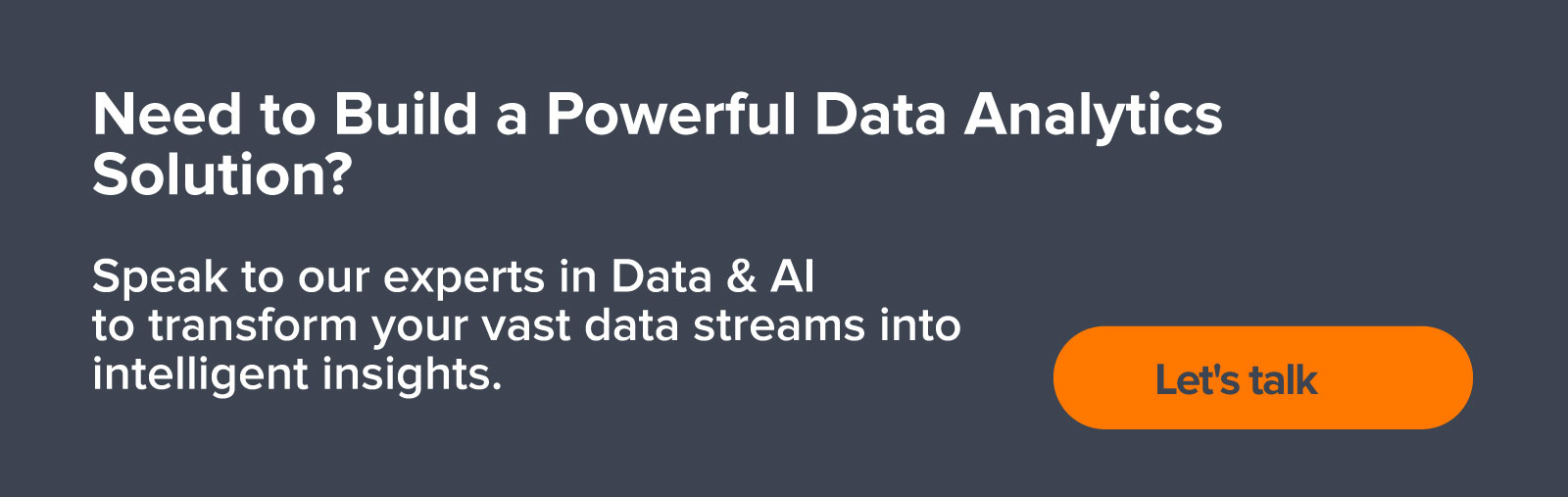
A similar profession existed a long time ago called a “data analyst.” Data analysts, however, are working with a predefined set of data, which is tied together, while a data scientist tries to build dependencies and new algorithms to get new insights.
Dealing with big data does not only require a tool and understanding of the business you are working in, but also a knowledge of data processing applied math, and programming, as there is no way to construct a tool that works with general data.
In 2016, there were many misleading messages about the difference between data analysis and machine learning. Their key point was that the results that you get from data analysis were repeatable, while the insights that you get from big data were foresighted and predictable. A data scientist builds a model based on data that he already has to help predict the future, while a data analysis talks about what happened in the past.
To sum up: if you want to get a benefit from big data, you need to do the following:
- Identify what data you want to collect and how it collates to insights you are looking for.
- Create data lake within the company where you are collecting all unstructured data.
- Hire a data scientist that can extract insights from big data.
- Create a data processing environment, where the data scientist can execute his/her models on a large scale.
- Implement visualization of insights and required actions using business intelligence on a continued basis.
Big data is a great source of information, but it does not have a magic wand that immediately provides insights. It needs to be analyzedmanually, and that takes not just time but also a smart and clear mind.
This opens up a huge opportunity for the outsourcing market, where more and more companies are hiring third parties to help them get insights into their businesses, by analyzing unstructured data that they own.