Interest in artificial intelligence (AI) has grown at a massive clip. ChatGPT and other large language models (LLMs) released since then have given the public a taste of AI capabilities. Machine learning (ML) and deep learning (DL) systems that also go into the AI umbrella have been deployed by businesses since the early 2000s.
Much of the recent hype has been driven by the growing discussion of the AI ROI. McKinsey estimated that generative AI models can add an equivalent of $2.6 trillion to $4.4 trillion globally in productivity gains. PwC pegged the number to $15.7 trillion in global economic output by 2030, more than India and China's combined GDP.
The modeled macro-impacts are certainly impressive, but organizations struggle to estimate the ROI of AI solutions on a company level. Among 700 AI adopters surveyed by Gartner, almost half indicated challenges with demonstrating the value of AI investments.
This post offers guidelines on how to approach AI ROI calculation best to quantify the impacts and value of AI investments.
How to Measure the ROI of AI: Selecting the Right Metrics
Proving the ROI of AI is, to an extent, as tricky as demonstrating the ROI of cloud migration. Estimating an AI system's total cost of ownership (TCO) is relatively easy, but demonstrating direct monetary savings or gains is hard.
Most AI impacts, such as process optimization, business model transformations, or new revenue enablement, will be indirect. Respectively, simple ROI formulas of dividing financial gains by investment costs won’t work.
A better way to measure the impacts and value of AI is by using proxy measures: technical performance and business success metrics.
Technical AI Performance Metrics
Evaluate AI model performance based on model quality, system quality, and business impact.
AI model quality metrics measure the model's performance in production in terms of speed, accuracy, and precision. For many AI use cases, models must show a high percentage of correct predictions or classifications, as well as millisecond latency for near-instant output. Performance above 80% is considered advanced. No ML, DL, or gen AI models score 100% on all quality metrics.
Papers with code curates an AI model benchmark database for various use cases: computer vision, natural language processing, general recommendations, time series predictions, and others. The models show varied performance on different tasks and datasets.
For example, the Co-DETR model for object recognition had the best performance on several Microsoft Common Objects in Context datasets with annotated image data. However, YOLOX showed better performance on datasets without predefined anchor boxes. This algorithm also works better for cases when you need to understand better the object shapes and instance segmentation, such as medical image analysis or defect detection on photos of industrial assets.
Benchmarks give a good sense of the models’ innate capabilities, but the actual results of your AI teams may differ from the scientific baselines due to data quality, availability, and augmentation methods.
AI system quality encompasses a broader range of metrics for evaluating the system’s key components: data pipelines, flow orchestration, resource consumption, interoperability with other systems, and overall robustness.
Metrics like availability (percentage of uptime), throughput (number of tasks, proceed per minute), and interoperability characteristics help better evaluate the effectiveness of solutions, running in productions. Tracking security and relevant compliance metrics is also important to ensure safe, bias-free, and ethical outputs that wouldn’t lead to regulatory troubles.
To select the right metrics for demonstrating the ROI of machine learning or generative AI solutions, always consider the project’s goals and wider business objectives. For example, minimizing false negative rates in an investment management application is more critical than improving its overall accuracy. On the other hand, you may want to prioritize accuracy for a specific type of medical image (e.g., radiography lung scans), rather than trying to increase model precision across multiple image types.
Sample Machine Learning and Deep Learning Metrics
Accuracy metrics indicate the percentage of correctly predicted/classified cases versus the total number of processed ones. Popular accuracy metrics include:
- Mean Squared Error (MSE), Mean Squared Error (MSE), and R-squared for regression logical models.
- Top-k Accuracy, F-1 score, and AU-ROC for classification models.
Precision as a standalone metric shows the ratio of true positive predictions vs the total predicted positives. You can get extra valuable data by also measuring:
- Weighted precision — an average precision across all classes, weighted by the number of true instances for each class — for predictive analytics models helps better understand how different classes affect the model precision and how the model performs when certain data classes are underrepresented.
- Mean average precision — mean of the average precision scores across all queries helps understand how recommender systems or search models rank and retrieve different information in response to user inputs. It tells how well the algorithm ranks relevant vs non-relevant items.
Recall (or model sensitivity) is the ratio of true positive predictions to the total actual positives, measuring the model’s ability to identify positive instances correctly. High recall is crucial for information retrieval, NLP, anomaly detection, and object classification models as their value hinges on their ability to detect positive instances. Helpful recall metrics include:
- Micro and macro recall
- Weighted recall
- Top-k recall
- False negative rate
Generative AI Performance Metrics
Several quality indexes help measure the quality aspects of generative AI models — BLEU, SuperGlue, and BIG-bench among others). Similar to ML/DL models, you should also set minimal acceptable accuracy thresholds and an acceptable error rate in outputs.
When it comes to quality output and content safety measurements, you can either rely on human feedback or fine-tune your system to self-test. Microsoft Azure AI Studio, for example, supports AI-assisted performance and quality evaluations of fine-tuned versions of GPT-4 and GPT-3.
Business Success Metrics for AI
Technical performance metrics demonstrate how well the AI system handles the assigned tasks. They help data science teams select the optimal model versions for deployment and monitor their performance in production. Monitoring is essential to prevent problems like data and concept drift, data leakage, and biased outputs among others.
The next step in estimating the ROI of AI is to correlate the model’s performance with specific business outcomes. Typically, businesses use proxy variables like customer satisfaction, productivity improvements, or new revenue enablement to showcase the impacts of AI projects.
Here are several sample business KPIs you can use to demonstrate the ROI of AI:
- Percentage of workflow automation. AI models start bringing value when they’re embedded into business workflows and aid (or fully automate) their execution. To demonstrate the impacts, you can show stakeholders how much of the previously manual (and low-value) processes have been fully or partially automated, leading to lower error rates and/or operational savings. Unilever, for example, fully automated quality checks of its Rexona deodorant production. With a new computer vision system in place, staff can be redeployed to more mission-critical tasks on the production floor, leading to greater operating efficiencies.
- Productivity gains. Intelligent task automation, with the help of AI systems, increases personal productivity. Microsoft conducted a study with early Copilot adopters across its product ecosystem. In almost all cases, they observed a significant increase in task execution speed. Copilot users completed tasks faster 26% to 73% of the time. Moreover, 72% of participants agreed that gen AI tools helped them spend less mental effort on mundane or repetitive tasks and 70% believed that Copilot increased their productivity. To showcase the benefits of AI systems, you can measure teams’ performance with and without such tools for a set of automated tasks.
- Service level improvements (e.g., customer service). AI solutions empower both your employees and customers to accomplish more tasks with higher speed, accuracy, and precision. This leads to improvements in metrics like higher customer satisfaction, higher retention, and overall engagement, which are relatively easy to track. Atlantic Health System, for example, measures the return on investment in AI through the pane of patient-focused metrics like length of stay, faster time to treatment, faster insurance eligibility verifications, and faster prior insurance authorizations.
- Adoption rates. A solution that generates no excitement among users will not last long on the market and fail to produce good returns. Therefore, tool adoption rates can be a good proxy measure of your AI ROI. GitLab Duo, a suite of AI tools for DevSecOps workflows, for example, offers convenient analytics dashboards to cross-correlate AI adoption rates among your team with performance-level improvements. Users can track metrics like lead time, daily deployment frequency, change in failure rate, and critical vulnerabilities, as well as see the metric evolution over time with greater product adoption rates across different teams and processes.
- New revenue enablement. Lastly, companies with a strong big data analytics function also quantify the impacts of AI solutions on revenue-related metrics like user base growth, conversion rate increases, customer lifetime value growth, and others. Such measurements, however, won’t be definite as many factors can impact user base growth apart from say new AI sales enablement tool adoption. The best option is to select several processes, where AI impacts will be the most apparent and then track respective revenue metrics. For example, you can evaluate the effects of AI-powered product recommendations by benchmarking the average order value for two cohorts — one receiving recommendations and the other not.
How to Maximize the ROI of AI
AI adoption requires high capital investments. Poor market fit, high operating costs, questionable business value thus risk becoming costly mistakes. Gartner estimates that by 2025, 90% of enterprise-gen AI deployments will slow down as costs exceed value, and 30% of these may be fully abandoned.
Companies that get the highest ROI from AI follow a series of best practices that enable a strategic approach to AI development and steady progress along the AI maturity curve.
Organize Your Data
Every type of AI algorithm requires ample data for model training, validation, or fine-tuning. Low-quality or siloed data is thus one of the biggest barriers to successful deployments. IBM estimated that organizations with strong data management practices get twice the higher ROI from its AI projects.
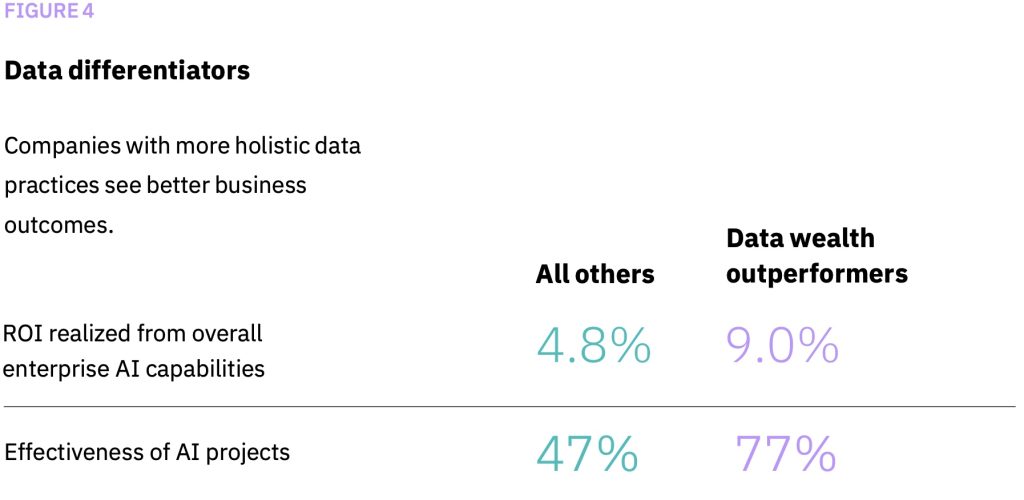
Source: IBM
Most businesses sit on massive data reserves. However, many of these insights are stashed across multiple business systems in different formats. You need to implement data pipelines to make this data usable for different AI projects.
A data pipeline includes an orchestrated number of steps — ingestion, processing, storage, and access — for transforming raw data from multiple business systems into datasets that can be queried with ad hoc tools and used for model training. Robust data pipelines and effective data governance enable fair, explainable, and secure AI solutions.
Focus on Quick Wins
Data quantity and quality will determine what AI use cases you can pursue to an extent. You should further limit your focus by evaluating the model’s utility.
AI is a versatile technology, but it isn’t necessarily the best solution to every problem. In some cases, a simpler data analytics model would do. In others: The cost of investment may substantially outweigh the potential gains.
To ensure strong ROI, look into AI use cases with a good market fit and proven feasibility. For example:
- AI in education has shown the most traction in learning personalization, automatic testing and grading, and student performance analytics.
- AI in healthcare increases the speed of analyzing medical imagery, drug discovery, and hospital resources management.
- AI in finance is having the greatest impact on fraud detection, personal finance management, and investing.
By prioritizing use cases with strong demand and high applicability, you can secure faster adoption and gain metrics for measuring the business impacts. With early quick wins, securing support for more complex projects with longer timelines and delayed ROI is easier.
Optimize Your Tech Stack
AI solutions development is an interactive process. On average, data scientists discard over 80% of machine learning models even before deploying them to production because of poor performance results in the sandbox environments and/or deployment complexities.
AI systems aren’t just expensive to develop; they also cost a pretty penny to maintain. ChatGPT, for example, is rumored to burn over $700K daily in misc operating and technology expenses. Over half of enterprises that have built large language models (LLMs) from scratch will abandon them by 2028 due to cost, complexity, and technical debt, according to Gartner.
To avoid such scenarios, you must create an effective MLOps process for developing (fine-tuning), testing, and deploying AI models to production. Short for machine learning operations, MLOps adapts software development best practices like continuous integration and delivery to AI model development. MLOps aims to bring greater predictability into model deployments through process automation and standardization.
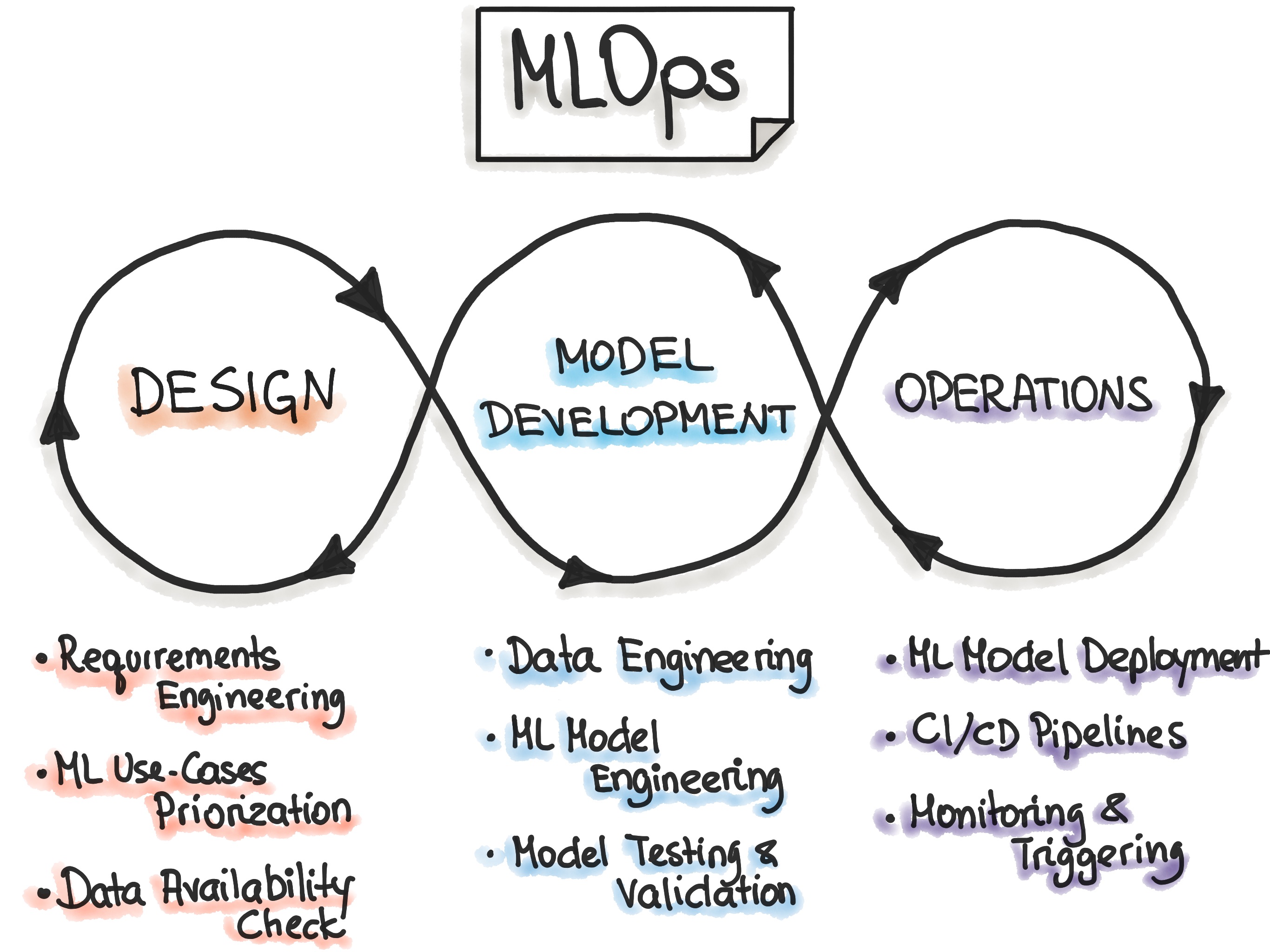
Source: MLOps
Teams with mature MLOps practices face fewer difficulties in implementing AI on a large scale. They’re also deploying new model versions faster, requiring less than a month to turn an idea into a new model, against an average of seven months for other teams.
Apart from enabling process efficiencies, MLOps also helps generate infrastructure cost savings. By implementing better infrastructure monitoring and automating workflows with Kubeflow, a popular open-source MLOps platform, Ntropy, a developer of custom LLMs for finance companies, reduced its cloud costs by 8X times.
The Final Element of Proven AI ROI: Great Talent
Cutting-edge AI algorithms are built and operated by people. Access to expert machine learning, AI, and computer vision talent is critical to ensuring successful implementation and tangible ROI.
Likewise, AI knowledge-sharing is essential for AI tools adoption by end-users — employees or customers. IBM found that companies that offer technical training and promote knowledge sharing achieve 2.6X higher ROI than others.
That said, exceptional AI engineering talent is extremely hard to find and cultivate, with 63% of businesses admitting shortages are in AI and machine learning. An alternative approach to engaging in talent wars is partnering with external vendors. Svitla Systems has been delivering end-to-end AI implementation support to businesses seeking to become market leaders.
Contact us to learn more about our approach to strategic collaboration and ways we could help your business with strategizing and claiming ROI from AI.